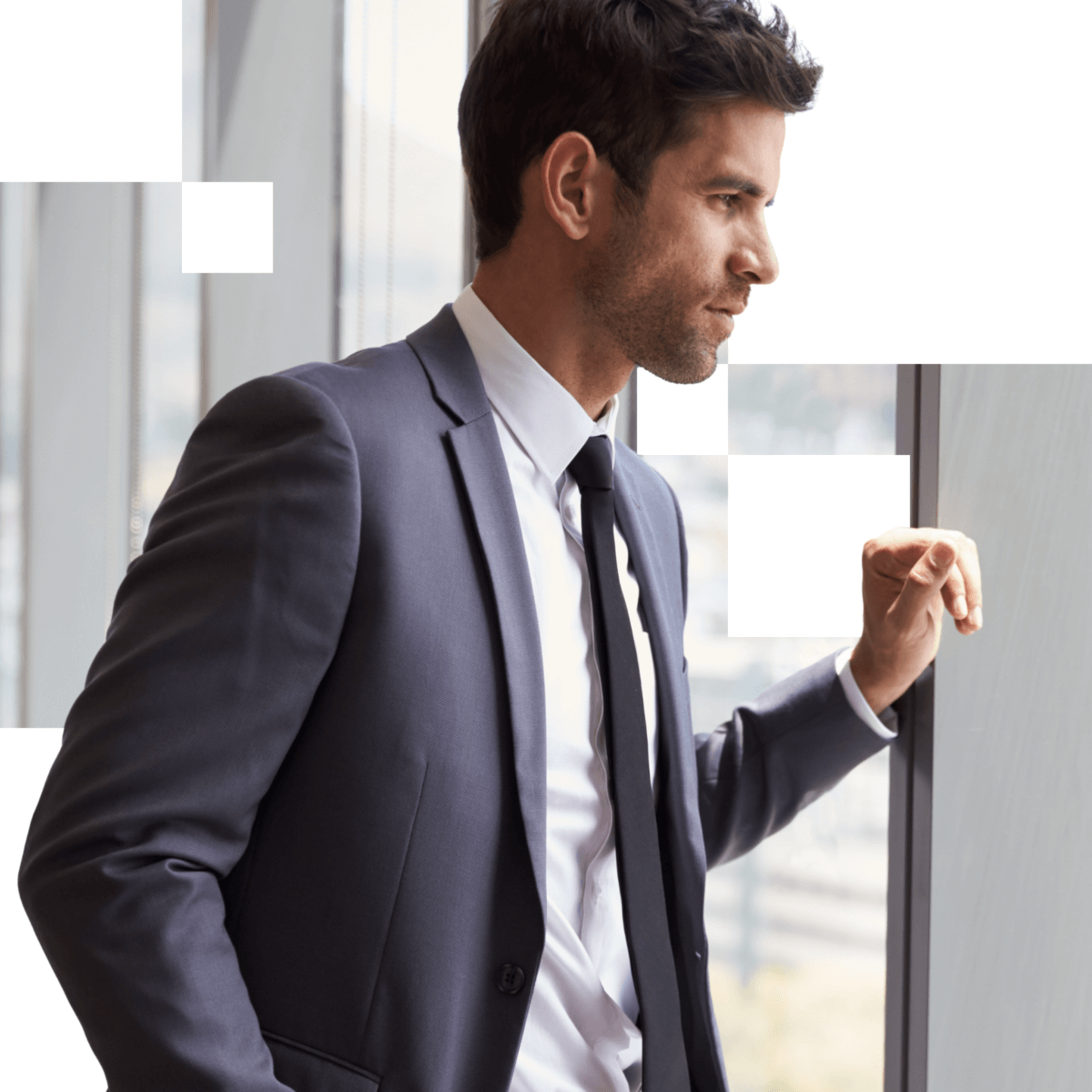
How to Prevent an API Breach
According to analyst firm ESG, 92% of cybersecurity professionals have experienced at least one API security-related incident in the last 12…
{ "term_id": 297, "name": "John Natale", "slug": "john-natale", "term_group": 0, "term_taxonomy_id": 297, "taxonomy": "wpx-authors", "description": "", "parent": 0, "count": 31, "filter": "raw" }
Key Takeaways
In the world of data management and cybersecurity, data classification plays a crucial role in determining how different types of information should be protected. It involves categorizing data based on its sensitivity, importance, and level of security required.
Data classification involves sorting and grouping data according to its level of sensitivity, significance, and worth. This allows organizations to identify the necessary security measures for safeguarding their data. Labeling or tagging datasets enables efficient management and protection of valuable information assets.
The importance of effective data protection cannot be overstated. As cyber threats continue to evolve and become increasingly advanced, it is imperative to prioritize the identification and safeguarding of sensitive information from unauthorized use or access. One effective approach is to categorize data based on its level of sensitivity, allowing organizations to implement appropriate security measures to protect their assets.
Adhering to regulations is a critical concern for numerous industries. Diverse regulations dictate how specific forms of sensitive information should be managed and secured—for example, HIPAA for healthcare or GDPR for individual data privacy in Europe. Data classification helps organizations in complying with these regulations by recognizing datasets that meet particular requirements. By ensuring compliance, organizations can evade legal consequences and foster trust among customers and stakeholders.
Effective data classification is essential for successful risk management. By evaluating potential risks related to various classifications, companies can concentrate on safeguarding valuable information and efficiently allocate resources. This allows them to prioritize protecting crucial assets that could result in significant consequences if exposed or jeopardized.
A major benefit of well-classified datasets is the efficient management of data throughout its lifecycle. This includes careful handling at each stage, such as secure storage during retention periods and appropriate disposal techniques when expiration dates are reached.
Ultimately, effective data classification enables organizations to gain a comprehensive understanding of their data landscape and prioritize security measures accordingly. Through accurate identification and categorization of sensitive information, businesses can safeguard their assets, comply with regulatory requirements, manage risks effectively, optimize resource allocation, streamline data management processes, and foster secure collaboration. These actions collectively contribute to enhanced protection of valuable information in the modern digital landscape.
The classification of data is a vital consideration when it comes to data management, as it determines the appropriate level of protection for various types of information. This process involves organizing data according to its sensitivity, significance, and necessary level of safeguarding. Let’s explore some common types of data classification:
Categorizing data based on specific criteria, such as sensitivity, confidentiality, or importance, is a crucial step in managing and organizing large amounts of information. This process, known as data classification, offers many advantages for organizations. However, it also presents significant difficulties that must be resolved for successful implementation.
Effective data classification is essential for organizations to manage and protect their valuable information. By implementing best practices, organizations can make sure that data is properly categorized, labeled, and protected according to its sensitivity. Here are some key best practices for effective data classification:
Data classification plays a pivotal role in abiding by compliance regulations such as GDPR by providing thorough handling of sensitive data. By categorizing data into different levels based on sensitivity, your organization can precisely identify and protect data under regulatory scrutiny.
This systematic approach enables efficient management and implementation of necessary safeguards, mitigating risks of non-compliance. Additionally, data classification facilitates targeted data analytics categories, enhancing insights while maintaining regulatory adherence. Classifying data mining systems helps organizations navigate complex regulations, building trust and accountability in their data handling practices.
The classification of data mining systems enhances cybersecurity by enabling tailored security measures based on data sensitivity. By categorizing data into different levels, your organization can identify vulnerabilities and implement appropriate safeguards, reducing the risk of data breaches and unauthorized access. This proactive approach makes sure that sensitive information is protected against cyber threats.
Noname excels in safeguarding against cybersecurity threats with advanced API securitysolutions. Request a demo of our API security platform to see how Noname empowers businesses to fortify their defenses and protect their valuable data assets.
Various tools and technologies are recommended for data classification, catering to diverse organizational needs. Software solutions like Microsoft Azure Information Protection, Symantec Data Loss Prevention, and Varonis Data Classification Engine offer robust capabilities for data categorization across different data classification levels.
Hardware solutions such as dedicated appliances and storage systems with built-in classification features further enhance data security. Additionally, incorporating security testing into your classification process protects the effectiveness of implemented measures, safeguarding against potential vulnerabilities and threats. Explore these options to establish comprehensive data classification frameworks aligned with your security objectives.
Periodic data review and reclassification is vital to ensure alignment with evolving business dynamics, legal mandates, and data sensitivity. The frequency of reclassification depends on factors such as the pace of business operations and regulatory changes.
Generally, data should be reclassified at regular intervals, ideally annually or semi-annually, to accommodate shifts in organizational priorities and compliance requirements. This proactive approach makes sure that data classification levels remain accurate and reflect current circumstances. Incorporating APIs into the reclassification process streamlines updates across systems, enhancing efficiency and maintaining data integrity amid dynamic environments.
Experience the speed, scale, and security that only Noname can provide. You’ll never look at APIs the same way again.